The Role of AI in Predictive Analytics
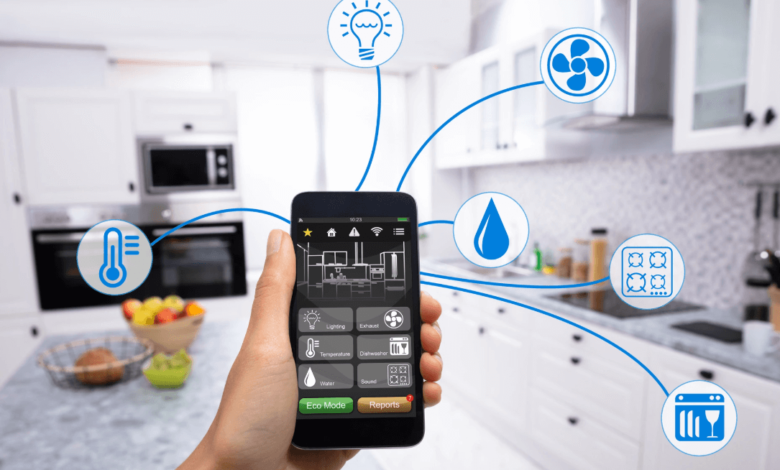
The integration of artificial intelligence into predictive analytics represents a transformative shift in how organizations approach data interpretation and decision-making. By leveraging sophisticated algorithms and data mining techniques, AI enhances the ability to discern patterns within historical data, thus enabling more accurate forecasts across various sectors. However, the adoption of these technologies is not without its challenges. As we explore the complexities and advantages of AI in this context, it becomes essential to consider not only the potential benefits but also the obstacles that organizations must navigate to fully harness this capability.
Understanding Predictive Analytics
Predictive analytics encompasses a suite of statistical techniques and machine learning algorithms designed to analyze historical data, identify patterns, and forecast future outcomes with a high degree of accuracy.
By examining data trends, practitioners can enhance model accuracy, enabling informed decision-making.
This analytical approach empowers organizations to anticipate changes, optimize strategies, and ultimately pursue greater autonomy in their operations and planning.
AI Technologies in Predictive Analytics
The integration of AI technologies into predictive analytics significantly enhances the capabilities of traditional statistical methods, allowing for more sophisticated data processing and improved forecasting accuracy.
Key AI technologies include:
- Machine learning algorithms for pattern recognition
- Advanced data mining techniques
- Neural networks for deep learning
- Natural language processing for unstructured data
- Predictive modeling tools for scenario analysis
These innovations drive more informed decision-making.
Benefits Across Industries
Across various industries, the application of AI in predictive analytics has led to transformative enhancements in operational efficiency, risk management, and strategic planning.
Healthcare applications improve patient outcomes through predictive diagnostics, while retail forecasting optimizes inventory levels.
Financial modeling enhances investment strategies, and manufacturing optimization streamlines production.
Moreover, leveraging customer insights refines marketing strategies, driving targeted engagement and increasing profitability across sectors.
Challenges of AI Implementation
Numerous challenges hinder the effective implementation of AI in predictive analytics, ranging from data quality issues to the integration of complex algorithms within existing systems.
Key considerations include:
- Ensuring accurate and clean data
- Mitigating algorithm bias
- Achieving interoperability with legacy systems
- Addressing compliance and ethical concerns
- Managing the skill gap in data science expertise
These factors collectively impact the successful deployment of AI technologies.
Also read: The Rise of Smart Appliances in the Home
Conclusion
In summary, the integration of artificial intelligence into predictive analytics represents a transformative advancement in data-driven decision-making.
By harnessing sophisticated algorithms and data mining techniques, organizations can navigate the complexities of future uncertainties with enhanced precision.
While challenges persist in the implementation of these technologies, the potential to unlock unprecedented insights across various sectors remains tantalizing.
Embracing this evolution promises not only to refine strategies but also to illuminate pathways toward more informed and agile business practices.